Smarter Transportation Systems Through Data-Driven Insights
Provided the right information is available, the use of data analytics is bound to bring a revolutionary change to the transport industry. This transformation goes beyond a shift in technology; it is a complete reimagination of transport systems. Imagine a world where traffic is controlled, public transport is always on time, and delivery logistics are executed with unmatched accuracy, all because of data. As the world continues to urbanize, data analytics will play an even bigger role in coming up with adequate and sustainable solutions for transportation problems. This article focuses on an astonishingly intelligent future, identifying which methods of data manipulation can lead to more intelligent transportation systems with their corresponding difficulties.
The integration of data within transport planning and operations creates a variety of options to choose from. Real-time and historical data analytics can help cities enhance their transport networks. This article focuses on the endless possibilities of data and how its application has driven change. In the future, these elements will need attention not only from policymakers but from the entire public using public transport as a service. These sections will explain the goals for the data, outline current accomplishments, identify gaps, and foster changes that will advance transportation systems.
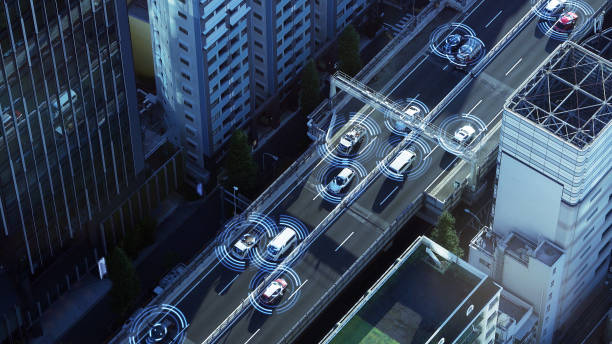
The Role of Data in Modern Transportation
In modern transport systems, data serves as the backbone of operational processes. Smart traffic lights and advanced scheduling for public transport enable organizations to base their decisions on data rather than estimates. Big data analytics enables planners to monitor and evaluate limitless parameters associated with transportation efficiency. For real-time data collection, IoT sensors and mobile applications are important technologies. The integration of such technologies by cities expands the opportunities for improving transport service delivery.
Gathering Real-Time Data
With the current advancement in technology, the collection and analysis of data can be done in real time. Traffic, location, and passenger data can be collected in real time through the integration of IoT sensors in vehicles and public infrastructures. Mobile users can be updated in real time, public transport routes can be changed, and traffic light timings can also be modified. These consist of the following techniques:
Sensors (IoT)
Mobile applications
Data collection via GPS
By collecting traffic data in real time, it is plausible for traffic to flow more smoothly, waiting times to decrease, and riders to be more satisfied with all forms of transport. Also, it is possible to ensure that decisions made by authoritative figures based on past data remain reasonable over time. The connection between modern live data and historical data tells the entire story of the transport system, which is important for control and planning activities.
Analyzing Historical Data
Furthermore, the analysis of transportation data is just as critical as the review of the sector’s historical data. Over the years, cities have accumulated a large amount of transportation data, which enables them to identify patterns. Previous traffic data illustrates which times of the day are busiest and how resources should be distributed. Evaluating the use of public transit by measuring the volume of passengers and their apparent preference for a bus system over a certain time frame provides valuable insights. With this insight, cities can proactively manage the peak times of public transport usage.
This analysis can reveal hidden obstacles that, if left unresolved, can propagate systemic issues. Here is how historical data can be applied:
- Estimating public transportation bus/train usage
- Studying variations of traffic volume in given seasons
- Spotting specific locations that are accident-prone
Following this data perpetually can improve policies in transportation, resulting in increased safety and efficiency. However, the most critical piece of this puzzle is contemporaneous data integrated with historical data regarding the trends and movements of transportation.
Case Studies of Effective Data Utilization
Transportation systems have numerous case studies to work with. Cities across the globe have begun experimenting with various data-centric methods, resulting in numerous advances. The effects of these implementations are analyzed from a real-life perspective, and the outcomes are, in fact, positive.
City | Data Utilization | Impact |
---|---|---|
Amsterdam | Smart Traffic Management | Reduced congestion and optimized traffic signals |
New York | Predictive Analytics for Public Transit | Improved bus and subway schedules |
Curitiba | Integrated Bus Rapid Transit (BRT) | Enhanced public transport efficiency |
These cities have used data not only to solve existing problems but also to anticipate future ones. Consider Amsterdam, which has implemented smart traffic management systems that have drastically reduced travel times and traffic congestion. In another example, New York has introduced predictive scheduling for subway and bus services, making travel far more convenient than before. These examples illustrate the clear impact that data-centric approaches have on transforming transportation in a city.
Challenges in Implementing Data-Driven Transportation Systems
Nonetheless, addressing the challenges of smarter transport systems remains problematic. A data-driven approach has several restrictions that could make it impractical. An overarching limitation is the issue of privacy and security. As modes of transport become more integrated, there is a greater risk of compromising user-sensitive data through unintentional breaches. Organizations must design and enforce robust measures to ensure such protection.
Public opinion and regulatory compliance set another boundary amid rising concerns about how information is collected, stored, and retrieved. There is a reluctance among government and business data owners to release information in a transparent manner with clear consent. Moreover, a broader challenge is the integration of various data sources. Transportation data is produced by various actors such as public authorities, private companies, and individual users. Integrating these disparate data streams can be very costly.
Consequently, many organizations struggle to strike a balance between user safety and leveraging the information received. There is a need for multi-stakeholder collaboration to permit proper data integration while meeting ethical obligations and standards. Achieving these goals results in earning public trust while motivating citizens to adopt data-oriented methods for managing transportation.
Future Trends in Transportation Data Analytics
The transportation sector is predicted to change rapidly in the future, especially with the rise in data analytics. The introduction of autonomous vehicles is likely to be the single most impactful change within this area. These vehicles require an extensive amount of data to navigate effectively and securely. Furthermore, AI and machine learning are being developed to ensure that such vehicles can continually adapt to their environment. Additionally, there will be a shift in the means of transport towards cleaner and more sustainable vehicles powered by data analytics.
Investment aimed at increasing the efficiency and safety of autonomous systems is pointless without data. Self-driving cars rely on data from a multitude of devices to improve routes, reduce waiting times, and lower the number of accidents. These factors not only improve the functionality of transport but, with the integration of other projects, can minimize traffic congestion and pollution. However, these technologies still have a long way to go, and in the meantime, authorities will need to streamline data governance as well as technology regulations that ensure safety without hindering innovation.
Similarly, the growth of green transportation is closely tied to data. As cities seek solutions for reducing their carbon footprint, data can help enhance the existing electric vehicle infrastructure as well as offer new routes for public transportation systems. The connection between data analysis and the development of sustainable transportation is strong and is based on the hope for a future where both effective resource use and ecological care are prioritized.
Conclusion
Integrating data into existing transportation systems is necessary for further automation and enhancement. Cities are not only trying to fix existing issues but are also preparing novel and eco-friendly solutions by merging real-time data with historical data. While navigating modern transportation challenges, the available data can significantly enhance operational efficiency and improve user experience. Incorporating data into mobility systems is still largely unexplored, but it is clear that those who embrace these changes will become frontrunners in the world of transportation.
Frequently Asked Questions
- What are smart transportation systems?
Smart transportation systems use technology and data analytics to enhance efficiency, safety, and user experience.
- How does data improve traffic flow?
By analyzing real-time traffic data, cities can optimize signals and reroute vehicles, effectively reducing congestion.
- What technologies are commonly used in data-driven transportation?
Technologies include IoT sensors, GPS tracking, and mobile applications that gather and analyze transportation data.
- Can data-driven insights help reduce environmental impact?
Yes, optimizing routes, improving public transit efficiency, and promoting electric vehicle use all contribute to sustainability efforts.
- What challenges exist in implementing these systems?
Key challenges include data privacy concerns, integration of diverse data sources, and the need for public acceptance of technology.